An Improved Active Shape Model Based Aortic Segmentation Method for CT Images
An active shape model and CT image technology, applied in the field of medical image processing, can solve the problems of difficult segmentation and extraction of aortic regions, a lot of clinical experience, difficult rescue and other problems, and achieve accurate and reliable experimental results, good adaptability and robustness. Effect
- Summary
- Abstract
- Description
- Claims
- Application Information
AI Technical Summary
Problems solved by technology
Method used
Image
Examples
Embodiment Construction
[0024] Algorithm flow chart of the present invention is as figure 1 As shown, first select some samples from the CT scan image sequence of aortic patients to build a training set, and mark the aortic region in the sample with feature points; the marked points of each CT image constitute a shape vector, and then all the constructed The shape vector normalization registration of the shape vector; in order to simplify the calculation of high-dimensional data, the principal component analysis method is used to reduce the dimension of the registered data to determine the main sample components, and build a statistical shape model; then the data after dimension reduction is established Grayscale texture model; establish SVM classifier in the training process; use SVM classifier to calculate target contour probability P(i, j) in the set of marker points, allowing the evolution of the outline to be based on the model outline. The combination of optimization and target contour and mode...
PUM
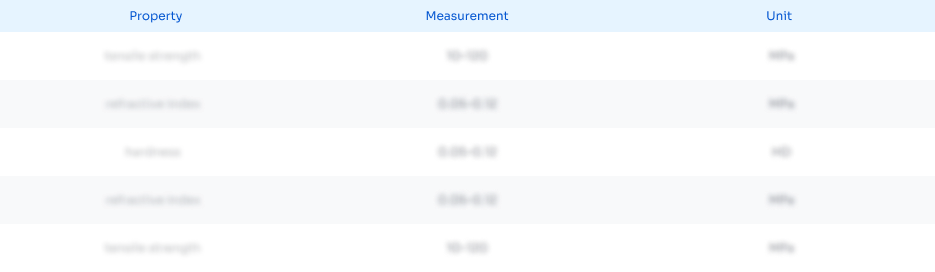
Abstract
Description
Claims
Application Information
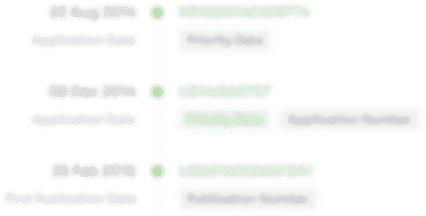
- R&D Engineer
- R&D Manager
- IP Professional
- Industry Leading Data Capabilities
- Powerful AI technology
- Patent DNA Extraction
Browse by: Latest US Patents, China's latest patents, Technical Efficacy Thesaurus, Application Domain, Technology Topic, Popular Technical Reports.
© 2024 PatSnap. All rights reserved.Legal|Privacy policy|Modern Slavery Act Transparency Statement|Sitemap|About US| Contact US: help@patsnap.com