Monocular unsupervised depth estimation method based on context attention mechanism
A technology of depth estimation and attention, which is applied in the fields of image processing and computer vision, can solve problems such as the inability to guarantee the sharpness of depth edges and the integrity of the fine structure of depth maps, the inability to obtain long-distance correlations, and poor quality depth estimation maps. Achieve good scalability, easy construction, and fast operation
- Summary
- Abstract
- Description
- Claims
- Application Information
AI Technical Summary
Problems solved by technology
Method used
Image
Examples
Embodiment Construction
[0026] The present invention proposes a monocular unsupervised depth estimation method based on the contextual attention mechanism, which is described in detail in conjunction with the drawings and embodiments as follows:
[0027] The method comprises the steps of;
[0028] 1) Prepare initial data:
[0029] 1-1) Evaluate the invention using two public datasets, KITTI dataset and Make3D dataset;
[0030] 1-2) The KITTI data set is used for training and testing of the method of the present invention. It has a total of 40,000 training samples, 4,000 verification samples, and 697 test samples. During training, the original image resolution is scaled from 375×1242 to 128×416. The length of the input image sequence is set to 3 during network training, and the middle Frames are target views, other frames are source views.
[0031] 1-3) The Make3D data set is mainly used to test the generalization performance of the present invention on different data sets. The Make3D dataset has ...
PUM
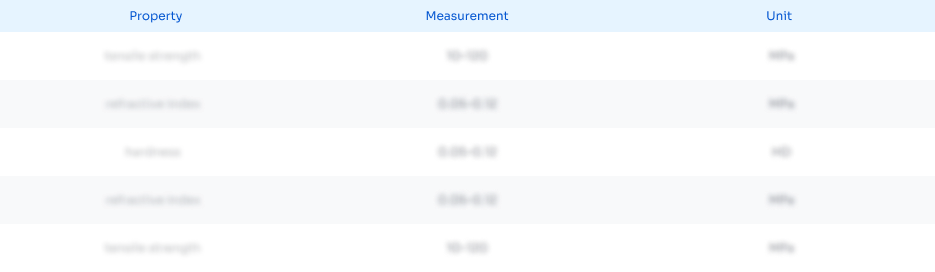
Abstract
Description
Claims
Application Information
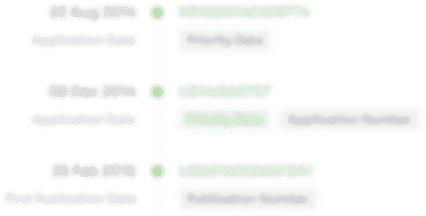
- R&D Engineer
- R&D Manager
- IP Professional
- Industry Leading Data Capabilities
- Powerful AI technology
- Patent DNA Extraction
Browse by: Latest US Patents, China's latest patents, Technical Efficacy Thesaurus, Application Domain, Technology Topic, Popular Technical Reports.
© 2024 PatSnap. All rights reserved.Legal|Privacy policy|Modern Slavery Act Transparency Statement|Sitemap|About US| Contact US: help@patsnap.com