Self-adaptive optical correction method and system based on convolutional neural network
A convolutional neural network and adaptive optics technology, applied in the field of optics, can solve the problems of difficult application of adaptive optics system and slow convergence speed, and achieve the effect of low cost and high system bandwidth
- Summary
- Abstract
- Description
- Claims
- Application Information
AI Technical Summary
Problems solved by technology
Method used
Image
Examples
Embodiment 1
[0038] see figure 1, in an embodiment of the present invention, an adaptive optics correction system based on a convolutional neural network, including a wavefront corrector 1, a wavefront corrector drive circuit 2, a main control computer 3, an optical beam splitter 4 and an imaging lens 5 , the function of the deformable mirror 8 here is to reflect the distorted wavefront to be corrected, which actually belongs to a part of the wavefront corrector 1, and an imaging lens 5 is arranged at one side of the wavefront corrector 1, and the The distorted wavefront to be corrected reflected by the front corrector 1 is incident into the imaging lens 5, and one side of the imaging lens 5 is provided with a focal plane CCD6 and a defocused plane CCD7. At the focus position, the distorted wavefront to be corrected by the imaging lens 5 is divided into two paths by the beam splitter 4, one of which is focused on the focal plane CCD6 by the focusing lens, and the other is focused by the fo...
Embodiment 2
[0040] see Figure 2-3 , Embodiment 1 has described the adaptive optics correction system based on convolutional neural network in detail. This embodiment mainly describes the adaptive optics correction method based on convolutional neural network. Specifically, the method includes the following steps :
[0041] S1, based on the convolutional neural network to train the convolutional neural network model driven by the distorted far-field light intensity and the wavefront corrector, use the convolutional neural network model to directly obtain the driving signal of the wavefront corrector according to the input light intensity, and use the drive The signal controls the wavefront corrector to generate a deformation conjugate to the wavefront to be corrected to correct the aberration of the incident wavefront;
[0042] Specifically, the construction method of the convolutional neural network model includes the following steps:
[0043] (1) Using the Zernike coefficient {a 1 ,a...
Embodiment 3
[0076] On the basis of Embodiment 2, this embodiment also adopts the stochastic gradient descent algorithm in the training of the convolutional neural network model, and learns the parameters of the established convolutional neural network by continuously reducing the function value of the loss function, specifically of:
[0077] The loss function is expressed as:
[0078]
[0079] In the formula, y i For the real Zernike coefficient, y_predicted i is the predicted Zernike coefficient.
[0080] The stochastic gradient descent method used in the training of the convolutional neural network model refers to the use of the samples in each iteration to learn parameters and updates, and the learning parameters and updates of each generation can be expressed as:
[0081] V i+1 =μV t -α▽loss(W i );
[0082] W t+1 =W t +V t+1 ;
[0083] In the formula, t is the number of iterations, W t is the parameter at time t, V t is the increment at time t, α is the learning rate, μ ...
PUM
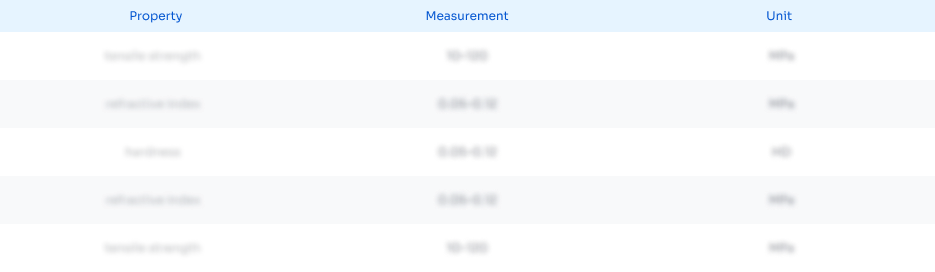
Abstract
Description
Claims
Application Information
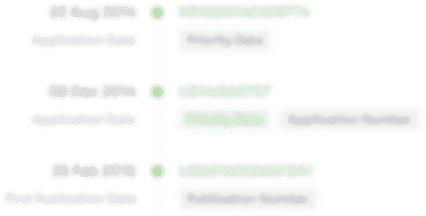
- R&D
- Intellectual Property
- Life Sciences
- Materials
- Tech Scout
- Unparalleled Data Quality
- Higher Quality Content
- 60% Fewer Hallucinations
Browse by: Latest US Patents, China's latest patents, Technical Efficacy Thesaurus, Application Domain, Technology Topic, Popular Technical Reports.
© 2025 PatSnap. All rights reserved.Legal|Privacy policy|Modern Slavery Act Transparency Statement|Sitemap|About US| Contact US: help@patsnap.com