Method for establishing perioperative period risk prediction model for coronary artery bypass transplantation
A coronary artery and risk prediction technology, applied in the field of biomedicine, can solve the problems of inaccurate prediction of in-hospital mortality, overestimation of mortality, and inaccurate prediction of population mortality
- Summary
- Abstract
- Description
- Claims
- Application Information
AI Technical Summary
Problems solved by technology
Method used
Image
Examples
Embodiment 1
[0028] From 2010 to 2019, 3659 domestic patients with complete clinical data who received coronary artery bypass grafting due to heart failure were collected as modeling research objects. In view of the completeness of the patient data and the actual collection results, the sorted patients include: gender, Hyperlipidemia, brain natriuretic peptide, thyroid function, hemoglobin, alanine aminotransferase, hypertension, body mass index, myocardial infarction, diabetes, cardiovascular stent implantation, elevated serum creatinine, cardiac surgery, smoking history, peripheral Arterial disease, cerebrovascular events, preoperative critical state, CCS4 grade, preoperative atrial fibrillation or atrial flutter, NYHA (heart failure) cardiac function III or IV, left ventricular ejection fraction (35%450pg / ml, between 50 and 75 years old, BNP>900pg / ml, over 75 years old, BNP More than 1800pg / ml; thyroid function, whether there is a history of abnormal thyroid; hemoglobin, with 140mmHg or ...
Embodiment 2
[0030]For the modeling group in Example 1, a univariate analysis was performed to analyze the relationship between each of the 25 initial risk factors and perioperative mortality, and the risk with a p value of 5% was screened. Factors, the relevant risk factors screened by the conditions are: gender, hyperlipidemia, brain natriuretic peptide, hemoglobin, elevated alanine aminotransferase (ALT), body mass index, history of myocardial infarction, diabetes, elevated serum creatinine , previous cardiac surgery, cerebrovascular events, medical history of abnormal thyroid function, preoperative critical condition, NYHA cardiac function class III or IV, left ventricular ejection fraction (35%<LVEF<45%, LVEF≤35%), combined valve surgery And combined with 17 risk factors of aortic surgery, the statistical analysis used was completed using SPSS20.0.
Embodiment 3
[0032] For the 17 related risk factors in step (2), use logistic regression analysis to judge multi-factor collinearity, remove collinear variables, and remove variables including hyperlipidemia, hemoglobin, body mass index, history of myocardial infarction, diabetes, and cerebrovascular events Seven risk factors, including preoperative critical state, were obtained, including: gender, elevated alanine aminotransferase (ALT), brain natriuretic peptide, history of abnormal thyroid function, previous cardiac surgery, elevated serum creatinine, cardiac The 10 final risk factors (11 independent variable factors), including functional class III or IV, left ventricular ejection fraction, combined valve surgery, and combined aortic surgery, are shown in Table 1 with their weights.
[0033] Table 1 Regression coefficients of risk factors
[0034]
[0035] By including: gender, elevated alanine aminotransferase (ALT), brain natriuretic peptide, history of abnormal thyroid function, ...
PUM
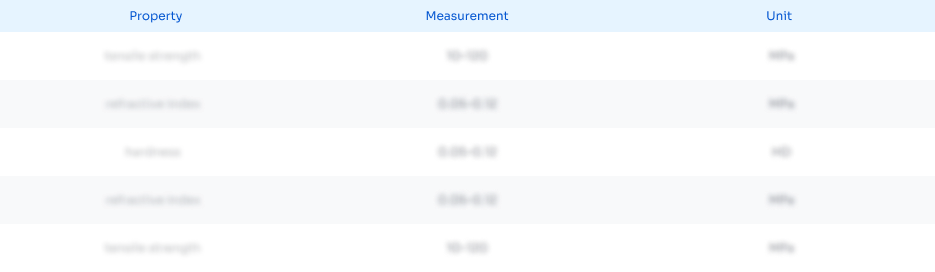
Abstract
Description
Claims
Application Information
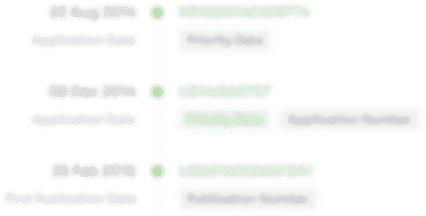
- R&D Engineer
- R&D Manager
- IP Professional
- Industry Leading Data Capabilities
- Powerful AI technology
- Patent DNA Extraction
Browse by: Latest US Patents, China's latest patents, Technical Efficacy Thesaurus, Application Domain, Technology Topic, Popular Technical Reports.
© 2024 PatSnap. All rights reserved.Legal|Privacy policy|Modern Slavery Act Transparency Statement|Sitemap|About US| Contact US: help@patsnap.com