A method for intelligent assisted identification for diseases like pancreatic cancer and pancreatitis
A technology for pancreatitis and pancreatic cancer, applied in the field of intelligent auxiliary identification of pancreatic cancer and pancreatic inflammatory diseases, can solve the problems of time-consuming and labor-intensive, ignoring the improvement of other modal image performance, poor feature representation and generalization ability, etc. , to avoid uncertainty
- Summary
- Abstract
- Description
- Claims
- Application Information
AI Technical Summary
Problems solved by technology
Method used
Image
Examples
Embodiment 1
[0061] The invention discloses an intelligent auxiliary identification method for pancreatic cancer and pancreatic inflammatory diseases. The specific steps are as follows:
[0062] 1) Reading of multi-modal images, and performing gray scale normalization operations;
[0063] 2) Image preprocessing, denoising, registration and other operations are performed on the normalized image obtained in step 1), to obtain a multimodal image with a uniform sampling interval of improved quality, and then perform image fusion;
[0064] 3) According to the multi-modal image and fusion image obtained in step 2), an experienced radiologist draws a rectangle to include the region of interest in the single-mode or fusion image with clear pancreatic structure, that is, select the rectangular target area, And map this region of interest to other modal images, and save the region of interest as a natural image format such as .png, .bmp, etc. that can be recognized by the subsequent classification n...
Embodiment 2
[0106] figure 2 The image in the middle is provided by Shanghai Changhai Hospital, taking PET / CT as an example to illustrate the image fusion process. First, the registered PET image a) and CT image b) are fused into a pseudo-image c), and then transformed into a grayscale image d) through grayscale transformation, so as to fuse the information of the two modalities together , to perform follow-up processing, or directly perform subsequent processing on the pseudo-image. The construction method of the above-mentioned pseudo-image is to use CT images of different HU value ranges as the two channels of the pseudo-image, and the PET image as the third channel.
Embodiment 3
[0108] An example of building a deep pyramid pooled convolutional neural network. Although the specific network structure of the deep pyramid pooled convolutional neural network for each modality image is different, they all include the following four parts:
[0109] 1) The input of any size image, the input image can be processed by decentralization, standardization, ZCA whitening, etc., making it easier to converge during training and speed up the training process;
[0110] 2) Construction of deep convolutional neural network (DCNN), which includes convolutional layer, pooling layer, BN layer, shortconnect, etc., by adjusting network depth, width, optimization algorithm, activation function, learning rate, etc. Excellent, so that it has the best feature extraction ability;
[0111] 3) Pyramid pooling layer, by introducing a pyramid pooling layer, the feature maps of different sizes generated by the convolutional neural network in 2) can be unified into a fully connected laye...
PUM
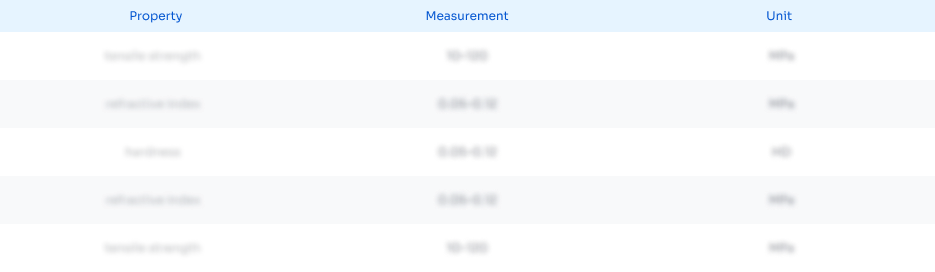
Abstract
Description
Claims
Application Information
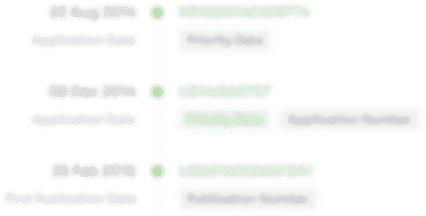
- R&D
- Intellectual Property
- Life Sciences
- Materials
- Tech Scout
- Unparalleled Data Quality
- Higher Quality Content
- 60% Fewer Hallucinations
Browse by: Latest US Patents, China's latest patents, Technical Efficacy Thesaurus, Application Domain, Technology Topic, Popular Technical Reports.
© 2025 PatSnap. All rights reserved.Legal|Privacy policy|Modern Slavery Act Transparency Statement|Sitemap|About US| Contact US: help@patsnap.com