A Raman spectral image data preprocessing method based on low-rank tensor algorithm
A technology of image data and Raman spectroscopy, which is applied in the field of spectral detection, can solve the problems of limited digital signal filtering means, long integration time, single means, etc., to reduce the signal acquisition time, the method is simple and easy, and the signal-to-noise ratio is improved. Effect
- Summary
- Abstract
- Description
- Claims
- Application Information
AI Technical Summary
Problems solved by technology
Method used
Image
Examples
Embodiment 1
[0039] A Raman spectral image data preprocessing method based on low-rank tensor algorithm, see figure 1 , the method includes the following steps:
[0040] 101: Convert the collected Raman spectral image data into the form of a third-order tensor, and construct a new low-rank third-order tensor by using tensor decomposition and reconstruction methods;
[0041] 102: Obtain the low-rank approximation tensor of the original data tensor through an iterative optimization algorithm and an optimal approximation algorithm;
[0042] 103: Build a database of noise distribution, judge whether the noise component in the original data conforms to the noise distribution based on big data statistics, if yes, perform step 104; if not, perform step 105;
[0043] 104: The low-rank approximation tensor at this time is the best low-rank approximation tensor. The best low-rank approximation tensor is the spectral information part in the original data, and a large amount of white noise and photon...
Embodiment 2
[0047] The scheme in embodiment 1 is further introduced below in conjunction with specific calculation formulas and examples, see the following description for details:
[0048] The embodiment of the present invention is mainly realized by a low-rank tensor algorithm. Taking the global low-rank decomposition method of a Raman spectral image as an example, the specific implementation method of the present invention is introduced in detail below:
[0049] Each spectrum in the Raman spectrum image has a great correlation, and according to the linear spectral mixture model, each spectrum can be composed of a small number of spectral end members, which proves that the real Raman spectrum image has low rank.
[0050] In actual measurement, noise will greatly destroy the low rank of the Raman spectrum image, and the noise in the Raman spectrum image can be separated by constructing the best approximation tensor of the collected data, thereby speeding up the acquisition speed of the Ra...
Embodiment 3
[0066] Combined with the following specific tests, figure 2 and image 3 The scheme in embodiment 1 and 2 is carried out feasibility verification, see the following description for details:
[0067] figure 2 (a) and (c) are raw data at Raman frequency shift 574.3cm -1 and 746.4cm -1 The two-dimensional Raman image at ; (b) and (d) are data processed by this method at a Raman frequency shift of 574.3cm -1 and 746.4cm -1 2D Raman image at . The measurement sample is a binary sample mixed with two samples. From the two-dimensional Raman spectrum images of the two characteristic peak positions of the two substances, it can be seen that the original data cannot distinguish the spatial distribution of the two samples at all. After processing by this method, the spatial distribution of samples can be clearly distinguished.
[0068] image 3 is the one-dimensional Raman spectrum of the processing result. Among them (a) is the original data, (b) is the data processed by this...
PUM
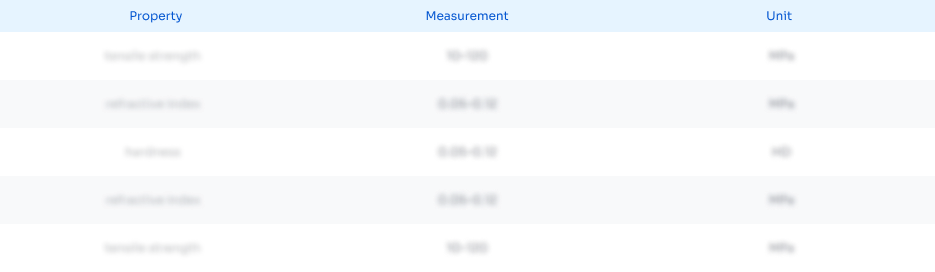
Abstract
Description
Claims
Application Information
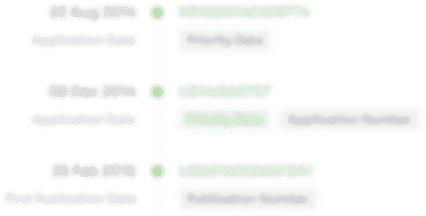
- R&D Engineer
- R&D Manager
- IP Professional
- Industry Leading Data Capabilities
- Powerful AI technology
- Patent DNA Extraction
Browse by: Latest US Patents, China's latest patents, Technical Efficacy Thesaurus, Application Domain, Technology Topic, Popular Technical Reports.
© 2024 PatSnap. All rights reserved.Legal|Privacy policy|Modern Slavery Act Transparency Statement|Sitemap|About US| Contact US: help@patsnap.com