Security early-warning model based on genetic wavelet neural-network
A technology of wavelet neural network and safety early warning, applied in the direction of biological neural network model, neural architecture, neural learning method, etc., can solve the problems of complex structure of wavelet neural network, incompatibility of real-time high efficiency, unsatisfactory convergence speed, etc., to achieve Simple structure, avoid accidents, reasonable design effect
- Summary
- Abstract
- Description
- Claims
- Application Information
AI Technical Summary
Problems solved by technology
Method used
Image
Examples
Embodiment 1
[0034] like figure 1 , 2 As shown, the safety warning model based on genetic wavelet neural network includes the following steps:
[0035] 1) Fuzzification of input data: Standardize and dimensionless the early warning indicators with the help of fuzzy mathematics and membership function;
[0036] 2) Determine the number of layers and the number of input, output and hidden layer nodes of the genetic wavelet neural network;
[0037] ①Establishment of a single BP wavelet neural network: determine the input and output neurons of the network. The input of the network is the endogenous variable of the system, and the output is the exogenous variable of the system. For the early warning of the production process subsystem and system, the early warning of the number of input nodes The number of indicators and the number of output nodes are divided into five levels of early warning according to the early warning level;
[0038] ② Design the number of hidden layers of the network: t...
Embodiment 2
[0058] The early-warning model of the present invention can be carried in a CD or chip to realize the safety early warning of the entire chemical production through the industrial computer. Due to the complexity of the chemical production environment, an anti-corrosion coating is provided on the surface of the industrial computer to prevent the industrial computer from being damaged and unable to alarm. The anti-corrosion coating is made of The following ingredients and parts by weight: 70-76.3 parts of water-based acrylic emulsion, 1-2 parts of ethyl naphthol, 8-18 parts of zinc powder, 1-4 parts of ditetradecyl alcohol ester, 0.1-0.2 parts of lithium chromate, poly 2-3 parts of ether diol, 0.6-1 part of polyacrylamide, 0.3-1.2 parts of dodecyl glucoside, 0.6-1 part of 2,6-di-tert-butyl p-cresol, 1- 5 parts, 1-2 parts of potassium dodecylbenzenesulfonate, 0.02-0.04 parts of antimony pentachloride, 0.4-1.5 parts of N-methylpyrrolidone, 0.3-0.5 parts of ricinoleic acid, 6-8.5 pa...
PUM
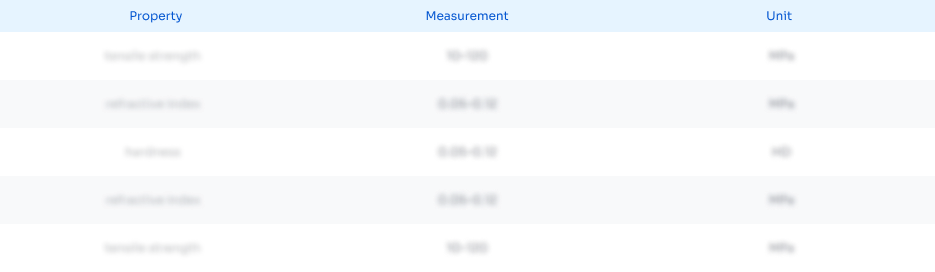
Abstract
Description
Claims
Application Information
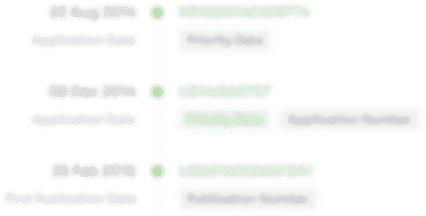
- R&D
- Intellectual Property
- Life Sciences
- Materials
- Tech Scout
- Unparalleled Data Quality
- Higher Quality Content
- 60% Fewer Hallucinations
Browse by: Latest US Patents, China's latest patents, Technical Efficacy Thesaurus, Application Domain, Technology Topic, Popular Technical Reports.
© 2025 PatSnap. All rights reserved.Legal|Privacy policy|Modern Slavery Act Transparency Statement|Sitemap|About US| Contact US: help@patsnap.com