Method and apparatus for normalization and deconvolution of assay data
a technology of assay data and normalization, applied in the field of processing data obtained from assay measurements, can solve the problems of limited adaptability to single step “homogeneous”, time-consuming and costly, and difficult multi-step workup and analysis procedures. , to achieve the effect of high sensitivity, low particle quantity, and high sensitivity
- Summary
- Abstract
- Description
- Claims
- Application Information
AI Technical Summary
Benefits of technology
Problems solved by technology
Method used
Image
Examples
example 1
Linear Normalization of Microarray Data
[0431]Application of the linear normalization method is demonstrated using artificial data generated in a Microsoft Excel® Spreadsheet, shown in the following Table 1. Data sets X and Y are representative of microarray expression intensity values acquired from two experiments. The actual values are from a random number generator. The first nine rows at the top of the table represent replicate control spots. The replicates were used to calculate a best-fit least-squares linear regression line, as described hereinabove. The last 14 rows of Table 1 represent replicate experimental spots. Column 3 of Table 1 was generated by the resulting linear transform equation. Table 1 includes ratios obtained both before and after normalization, along with their associated percentage change.
TABLE 1NormalizedNormalizedChange InData SetData SetData SetRatioRatioNormalizedXYY′(Y / X)(Y′ / X)Ratio68811071.191.5824%5470881.031.2920%6232220.470.32−47%446−240.09−0.35125%...
example 2
[0434]This example describes a study using microarray data from four slides: a set of two excellent-quality slides, referred to as “Ordered”, and another set of two poor-quality slides, referred to as “Disordered”. Each set of slides matches one another, feature-for-feature, and are expected to equally express across slides and within each slide. The slide layout depicted in FIG. 27 shows two slides, each having one array comprised of four sub-arrays. Each sub-array contains five classes of features replicated ten times in a column. The nomenclature used herein includes: Slides 0-1 (S0, S1); Groups 0-3 (G0, G1, G2, G3); and Features A-E. Each feature has an associated background value used in calculating an average background for each slide.
[0435]The purpose of analyzing two sets of data, Ordered and Disordered, is to demonstrate the degree to which this normalization technique affects high-variance data as compared to low-variance data. Normally, only high quality data should be us...
example 3
Bi-linear Normalization
[0455]Table B1 shows data sets “a” and “b” for each microarray feature C1 through C25.
TABLE B1Feature IDabC154471335C277533783C349952935C439531913C55015328C678306077C776453700C883622295C958371264C1044923823C1157734851C1294354318C1385762956C1462701617C1572232643C1694217261C173683508C1876614862C19299459C2061901119C2178993121C2267724486C233933105C24914175C2556363977
[0456]Data sets “a” and “b” are assigned to be the independent and dependent data sets, respectively, and arc each normalized and then converted to ratios. Then the data sets reverse roles and the process is repeated. These two results are depicted in Tables B2 and B3.
TABLE B2NormalizedControlDataNormalizedRatioFeature IDS0S1S1′[S0 / S1′]C15447133539101.39C27753378377011.01C34995293563880.78C43953191348050.82C5501532823502.13C678306077112540.70C77645370075731.01C88362229553961.55C95837126438001.54C104492382377630.58C115773485193550.62C129435431885301.11C138576295664201.34C146270161743461.44C1572232643593...
PUM
Property | Measurement | Unit |
---|---|---|
diameter | aaaaa | aaaaa |
diameter | aaaaa | aaaaa |
peak wavelength | aaaaa | aaaaa |
Abstract
Description
Claims
Application Information
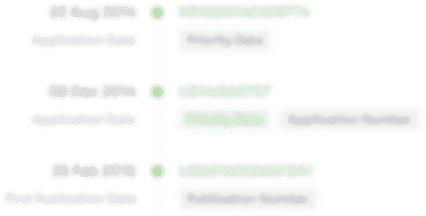
- R&D Engineer
- R&D Manager
- IP Professional
- Industry Leading Data Capabilities
- Powerful AI technology
- Patent DNA Extraction
Browse by: Latest US Patents, China's latest patents, Technical Efficacy Thesaurus, Application Domain, Technology Topic, Popular Technical Reports.
© 2024 PatSnap. All rights reserved.Legal|Privacy policy|Modern Slavery Act Transparency Statement|Sitemap|About US| Contact US: help@patsnap.com