Method for optimizing gradient titanium dioxide nanotube micro-patterns under assistance of machine learning
A machine learning, titanium dioxide technology used in the field of gradient TiO2 nanotube micropatterning
- Summary
- Abstract
- Description
- Claims
- Application Information
AI Technical Summary
Problems solved by technology
Method used
Image
Examples
Embodiment 1
[0035] Apply an active learning-based algorithm to search for boundary conditions and maximize TiO 2 Diameter range of nanotube micropatterns. After collecting a small dataset from bipolar electrochemical experiments, a machine learning algorithm builds a learned model and predicts the best outcome. The predictions are then tested experimentally, and the new results update the training dataset for the next active learning cycle. Such as figure 1 As shown, each active learning cycle consists of four steps: (1) acquire raw data from experiments; (2) define effective data boundaries with classification models; (3) data analysis / regression and prediction; (4) use grid Search for optimal experimental parameters for search and prediction.
[0036] First of all, we first pass a certain amount of experiments to accumulate raw data. Among the original data, the eigenvalues mainly include the specific parameters in the preparation process: voltage, reaction time, water bath temper...
Embodiment 2
[0040] In the active learning framework of Example 1, different classification and regression models are trained and generated respectively, and the optional models include linear models, polynomial models, decision tree models, support vector machine models, GBDT models or neural network models. Finally, the model with the highest accuracy is selected, the decision tree is used for classification, and GBRT (GBDT is used for regression algorithm) is used for regression processing. The selected model is established based on computer programming languages such as Python, Scikit-learning, xgboost, TensorFlow, and stored in one or more computer storage media. Decision tree is a decision analysis method for evaluating project risk and judging its feasibility by forming a decision tree to obtain the probability that the expected value of net present value is greater than or equal to zero on the basis of knowing the probability of occurrence of various situations. It is an intuitive...
Embodiment 3
[0042] A titanium foil with a thickness of 0.1 mm and a purity of 99.6% was cut into a shape of 38 mm × 10 mm, and then washed with an ultrasonic cleaner in the order of acetone, deionized water and ethanol for 20 min each. The titanium foil was then placed in air to dry. Preparation of TiO by Bipolar Electrochemical Anodization Based on the Best Protocol Recommended by Machine Active Learning 2 Nanotube micropatterns. Prepare 120mL electrolyte solution, which contains 0.75wt% ammonium fluoride, 10v% deionized water and 90v% glycerol, and stir evenly to obtain electrolyte solution. Fix the titanium sheet in the electrolytic cell described in step 1.3 (between the two platinum electrodes) with a high-temperature-resistant polyimide tape, and the distance between the metal titanium edge and the two platinum electrodes is 1 mm. Connect the positive and negative poles of the constant voltage power supply to the two platinum electrodes of the device to output a voltage of 160V. T...
PUM
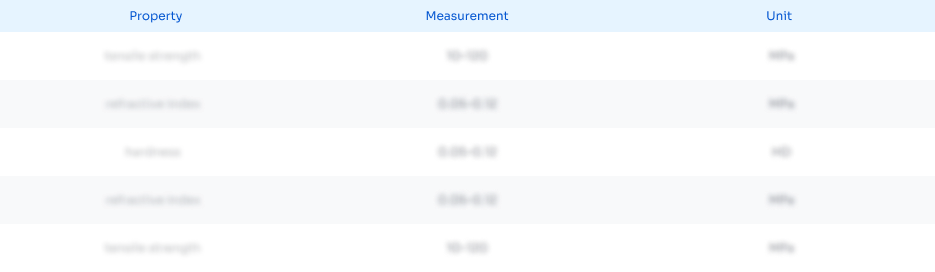
Abstract
Description
Claims
Application Information
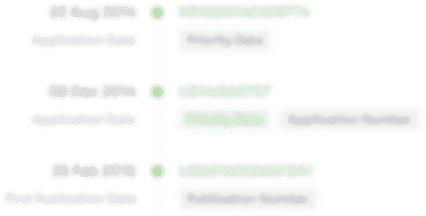
- Generate Ideas
- Intellectual Property
- Life Sciences
- Materials
- Tech Scout
- Unparalleled Data Quality
- Higher Quality Content
- 60% Fewer Hallucinations
Browse by: Latest US Patents, China's latest patents, Technical Efficacy Thesaurus, Application Domain, Technology Topic, Popular Technical Reports.
© 2025 PatSnap. All rights reserved.Legal|Privacy policy|Modern Slavery Act Transparency Statement|Sitemap|About US| Contact US: help@patsnap.com