Single-cell analysis and authentication method at high flux by combining Raman spectrum with artificial intelligence
A technology of Raman spectroscopy and artificial intelligence, applied in the field of cell analysis and identification, can solve problems such as broken cells, difficulty in realizing rapid analysis and identification of single cells, and inability to obtain information research on cell dynamics
- Summary
- Abstract
- Description
- Claims
- Application Information
AI Technical Summary
Problems solved by technology
Method used
Image
Examples
Embodiment 1
[0038] The embodiment of the present invention provides a high-throughput single-cell analysis and identification method combining Raman spectroscopy with artificial intelligence, including the following steps:
[0039] S1. Obtain the living cell fluid to be tested, and prepare the cell fluid into a single-cell array on the chip using microfluidic technology;
[0040] S2. Acquiring the Raman spectrum of the single-cell array and preprocessing the Raman spectrum data;
[0041] S3. Using the preprocessed data to perform model training, verification and inspection, and finally obtain an optimal model, and use the optimal model to identify and predict the preprocessed Raman spectral data.
[0042] in,
[0043] Step S1 is specifically:
[0044] Obtain the living cell liquid to be tested, and wash the cells in the obtained living cell liquid to be tested with saline or cell isotonic pressure solution for 3 times; the saline is 0.85% NaCl or NaCl suitable for the physiological conc...
Embodiment 2
[0062] 本发明实施例利用拉曼光谱仪采集获得Escherichia coli DH5α, Pseudomouasaeruginosa PAO1, Haloferax mediterranei ATu33sin, Sulfolobus islandicusE233S, Methanococcus maripaludis S2, Metallosphaera cuprina JCM 15769T,Acidianus brierleyi DSM 1651, Candida albicans SC5314, Cryptococcusneoformans JEC21, Saccharomyces cerevisiae W303-10D , S. arboricolusHZZt16L.1, S. kudriavzevii XS29L.2, S. mikatae FJSB44.3 and S. paradoxusCBS2908, a total of 14 microbial single-cell Raman spectra, and a total of 1301 Raman group data were obtained. These data were smoothed by convolution smoothing filter (Savitzky-Golay filter), baseline was removed by polynomial fitting method, and finally processed by vector normalization.
[0063] Input the preprocessed data into the built machine learning artificial intelligence algorithm model. The structural framework of the machine learning algorithm is shown in figure 1 . The structure contains 2 layers of convolutional layers, 2 layers of maximum pooling layers, and 1 ...
Embodiment 3
[0065] The embodiment of the present invention detects and analyzes the drug resistance of pathogenic microorganisms from clinic. We used a Raman spectrometer to obtain the Raman spectra of Aspergillus fumigatus (Aspergillus fumigatus) itraconazole-resistant strains and Candida albicans (Candida albicans) fluconazole-resistant strains from the clinic, as well as the wild strains of these two microorganisms. 723 Raman group data. The data pre-processing and machine learning training methods adopted are as in the above-mentioned embodiment 2. The result is as image 3 The results showed that the optimal model could well distinguish the drug-resistant strains of Aspergillus fumigatus from the wild strains, and also the drug-resistant strains of Candida albicans from the wild strains, with a sensitivity and specificity of 98%.
PUM
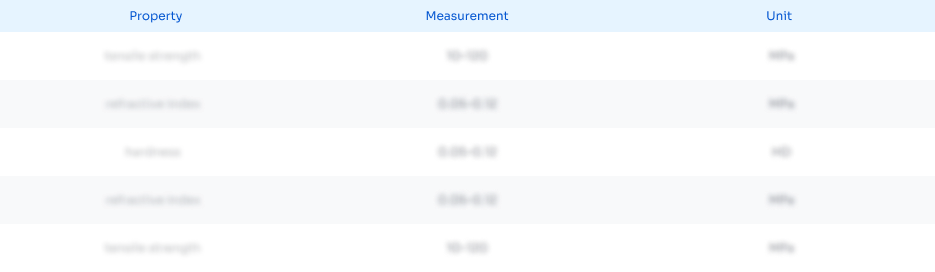
Abstract
Description
Claims
Application Information
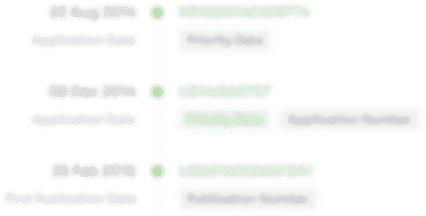
- R&D Engineer
- R&D Manager
- IP Professional
- Industry Leading Data Capabilities
- Powerful AI technology
- Patent DNA Extraction
Browse by: Latest US Patents, China's latest patents, Technical Efficacy Thesaurus, Application Domain, Technology Topic, Popular Technical Reports.
© 2024 PatSnap. All rights reserved.Legal|Privacy policy|Modern Slavery Act Transparency Statement|Sitemap|About US| Contact US: help@patsnap.com